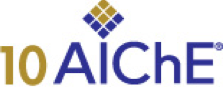
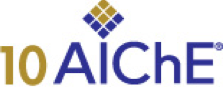
In recent years, there has been a marked surge in the search for alternative sources of energy that wean the world off of dependence on fossil fuels and reduce our carbon foot-print on this world. After a boom in U.S. corn-based ethanol in the early part of the 21st century, the interest has gradually shifted towards more viable sources of biofuels and biochemicals. Cellulosic ethanol, biodiesel, and syngas are examples of such fuels that are extremely attractive owing to the fact that the raw materials can be composed completely of “left-over” wastes of food crops and forest harvests that don't interfere with the human food chain and the natural ecosystem.
Even with increased research emphasis on biofuel and biochemical production technologies, large scale production is still hampered by many factors. Some of these factors that have received considerable interest in recent times include, cultivating more robust feedstock, genetically engineering enzymes and microbes that are more efficient and resistant to poisoning by by-products and improving process technologies that can render large scale production of biofuels a more profitable option for private entities. One area that has not received enough research interest in recent times is enterprise wide modeling and optimization in order to make the entire enterprise more competitive in the energy sector.
A sustainable enterprise is often defined as an enterprise that does not have a negative socio-environmental impact on the society. We further refine this definition to encompass not only the ability to positively impact the environment, but also maintaining such an impact through value creation and profitability. An enterprise is defined as being sustainable if it produces goods and services that benefit our environment and is able to preserve such an influence through continued growth.
Modeling an enterprise that produces renewable-based fuels and chemicals can provide valuable insight into the inter-play of the bioproduct supply-chain. This idea had been motivated by a recent surge in the research areas concerning supply-chain optimization and how cost-cutting measures throughout a supply-chain can render an otherwise sluggish enterprise, profitable. Our work adopts a decentralized approach for supply chain management in order to mirror actual enterprise architecture and to make the decision support tools function autonomously. In our work, a biorefining enterprise is characterized as an entity having three major layers in its functional hierarchy—the strategic planning layer, the tactical planning layer, and the production planning layer. Each layer is modeled with an optimization model. The strategic planning layer is formulated as a mixed integer capital budgeting and network design problem while the tactical planning layer is formulated as a resource allocation non-linear problem. The production planning layer consists of process simulations and optimization of three biorefining facilities with different operating capacities and product portfolios. What sets this work apart from other similar decision support frameworks is the formulation of the optimization models for the three planning layers that aim to exploit the synergy between different levels of planning in order to satisfy different enterprise objectives for varying time horizons. Disturbance rejection, that is, dealing with unexpected outcomes within a supply chain operation has also been emphasized in our approach.
The model for strategic planning represents the corporate level of an enterprise with an optimization model. The problem is a typical network and capacity design problem with added budgeting constraints and product and technology portfolio considerations. The objective function used in the model is the company's shareholder value and the optimization time horizon is five years with quarterly time increments. A forecasting module provides the model with demand and price forecasts for the five years. The model decision variables include asset acquisition, plant capacity design and increments, feedstock selection with seasonal crop yield considerations, quarterly production and inventory targets, market and market share selection, sales volumes, and distribution network design. The constraints and parameters introduced in the model include amongst others, mass balances on physical entities within the supply chain, raw material availability constraints, production and distribution capacity constraints, key performance indicators, tax subsidy considerations, and loan obligations. A two stage stochastic programming approach is used for optimization in order to deal with the inherent uncertainty in demand, yield and price forecasts. By doing a sensitivity analysis on such a model, one can determine key decision variables that can improve a company's shareholder value.
The tactical plan represents a resource allocation problem for a typical process enterprise. This model receives quarterly inventory targets, production levels, and sales volumes as constraints from the corporate level, and optimizes the supply chain model with the objective of maximizing profit. Key performance indicators such as inventory turnover are introduced in the model to measure the efficiency of the enterprise operation and shape the objective function. The time horizon for optimization is one quarter with biweekly time increments. Constraints and parameters used in the model include material balances similar in structure to the strategic planning model, and the minimum inventory stock levels (safety stock) required at all times. As an initial estimate, the sum of the biweekly demand forecasts for each market is forced to equal the quarterly demand for the strategic planning model and the biweekly prices for the product are formulated such that their average equals the product price input to the strategic planning model. Decision variables yielded by the model are production and inventory profiles for each facility, transportation quantities for each transportation link, and sales profiles for each market. More rigorous modeling for departments within the supply chain, such as the inventory and transportation department, can also be done to optimize their functioning.
The production plan assumes a given amount of resources and production targets and aims to achieve that while minimizing operating costs through heat integration. We use simulations of the process in this level to analyze the effects of the decisions of the other levels of planning on the production facilities. As a preliminary endeavor, the biorefining facilities simulated for were identical in process technologies used but had different operating capacities. The simulations were carried out in SuperPro and the process optimization was carried out in excel. The time horizon for the model is two weeks with daily time increments. Key decision variables yielded by the model are the operating points for the process units.
The three levels of planning and optimization have different objectives, time horizons, and time steps. This may lead to inconsistent results as plans from one layer may contradict plans generated by other layers. To accommodate these differences, we suggest the following implementation: (a) Implement the strategic optimization module every quarter with a five-year rolling time horizon and updated input parameters in order to take into account the realization of the of the tactical and production plans, an approach similar to a Model Predictive Control design, (b) Implement the tactical optimization module every two weeks but with a shrinking time horizon, and updated input parameters in order to take into account the realization of the first time step of the previous tactical plan, an approach similar to feedback-loop design, and (c) Implement the production simulation and optimization modules everyday with a shrinking time-horizon while trying to achieve the targets received from the tactical plan. Additionally, the constraint formulation for the tactical planning layer assumes a high level of importance in our framework. Since profit maximization should be, and is, the most important short-term goal, the constraints in the tactical planning layer should be structured in such a way that they allow the optimizer to search a complete solution space. Operational targets yielded by the strategic plan are used as constraints by the tactical planning model. We formulate the targets set by the strategic plan as “less than” or “greater than” constraints in order to provide a greater solution space for profit maximization.
Since information sharing is a key ingredient to achieve enterprise wide integration, a multi-agent system is envisioned to provide the system with the necessary framework to integrate the three levels of the enterprise. Future work will concentrate on providing the decision support system with real-time capabilities to ameliorate issues with information lag and bottlenecks within a supply chain. We will also investigate alternative network topologies, such as integration of biorefining technologies with current sugar and pulp and paper mills, along with the effects of R&D projects, mergers, and shutdown of facilities on the enterprise.
F&PD Members: Simply click on “click here to buy this archived webcast ” below and your ‘0’ price will appear in your shopping cart.
Preview Presentation
Presenter(s)
Language
Pricing
Individuals
AIChE Member Credits | 0.5 |
AIChE Pro Members | $15.00 |
Fuels and Petrochemicals Division Members | Free |
AIChE Graduate Student Members | Free |
AIChE Undergraduate Student Members | Free |
AIChE Explorer Members | $25.00 |
Non-Members | $25.00 |