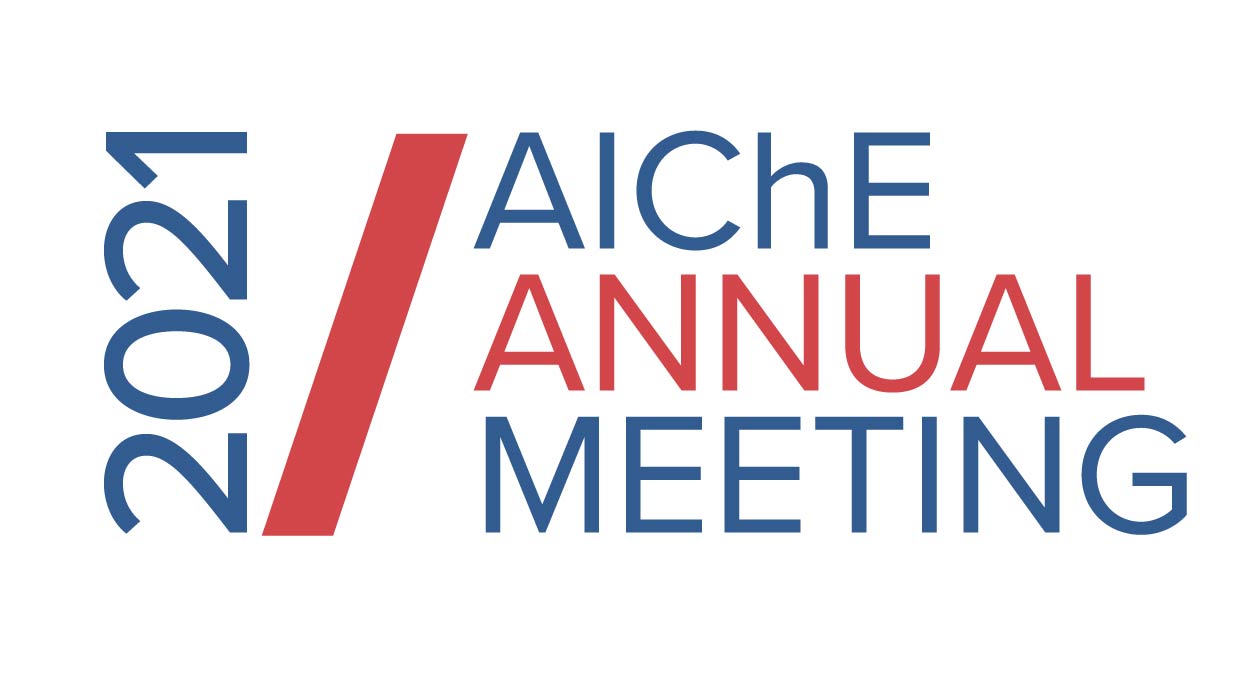
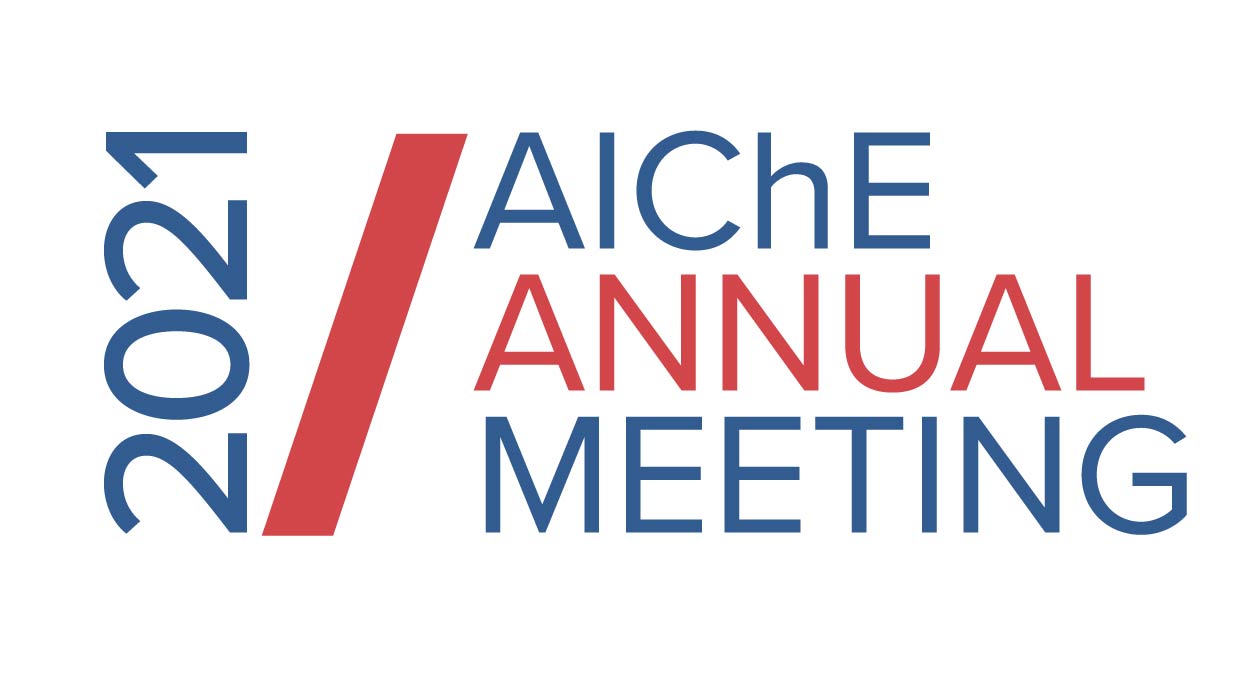
First, accurate segmentation of densely packed nuclei in fluorescence channels is critical for downstream tasks such as cell tracking, signal extraction and identity annotation. While many deep learning methods are available for 2D images, 3D segmentation methods for highly anisotropic images are not available. We combined a well-known DL framework for instance segmentation in 2D images [1] with optimal transport [2] based clustering to produce 3D segmentations in anisotropic images. Comparison against other methods on experimental and synthetic datasets show that our method is more accurate (5-8% higher F1 score) and more robust across a range of baseline cell signals and image noise levels (6-17% higher F1 score). Further DL method is 3.7 times faster than previous method.
Second, whole-brain imaging in freely moving worms is currently not widespread because of the requirement of custom designed microscopes with low-magnification behavior tracking and high-magnification fluorescence imaging channels [3-4]. However, commonly available microscopes in most laboratories do not have two light-paths for simultaneous low-magnification and high-magnification imaging. Thus, we developed and optimized a fast DL framework (30-644 times smaller model size and 5-8 times faster than previous methods) to directly predict the worm pose (skeleton) from high-magnification fluorescence channels, a key step in analyzing the behavior of animal. Fast inference of pose using only fluorescence channel enables worm-tracking thus eliminating the need of separate behavior channel. We show that predicted worm pose can be used for behavior analysis and cell-tracking in videos. Further, eliminating the need of custom microscopes will enable more labs to do whole-brain imaging in freely moving animals.
Third, image quality produced in microscope depends on acquisition parameters such as speed of imaging, laser power etc. For whole-brain imaging applications, high speed (>2 volumes per second) is necessary to capture fast activity transients, and low laser power is favorable to prevent photo-bleaching of fluorophores, light damage to worms etc. However high speed and low laser power acquisition decreases the signal-to-noise-ratio (SNR) in images. We developed a DL framework for restoring low SNR fluorescence images (acquired at low laser power/low exposure time) to high SNR images. Low laser power imaging eliminates photo-bleaching of fluorophores, light damage to worms and enables long-term neuron activity imaging across days. Image restoration can be performed on both GCaMP (green fluorescent protein for measuring neuron activity) and RFP (red fluorescent protein) channels. We show that restored images provide cleaner calcium signals compared to traditional de-noising methods (13-30% smaller mean-absolute error compared to clean trace). Additionally, restored images improve cell detection, tracking and identity annotation tasks.
Methods in our toolbox can be easily adapted for similar tasks in other organisms. These three methods will enable several applications e.g. accurate cell detection will improve cell tracking and signal extraction thus enabling faster analysis of datasets. Skeleton prediction method will enable more researchers to do whole-brain imaging in freely moving animals thus advancing the whole-brain research. Finally signal restoration method will enable newer studies such as long-term imaging of whole-brain circuits without photo-damage. Further cleaner neuron activity traces extracted from restored images will empower downstream statistical analysis such PCA, low-dimensional manifold projection etc.
- He, K., Gkioxari, G., Dollár, P., & Girshick, R. (2017). Mask R-CNN. 2017 IEEE International Conference on Computer Vision (ICCV), 2980â2988. https://doi.org/10.1109/ICCV.2017.322
- Cuturi, M. (2013). Sinkhorn Distances: Lightspeed Computation of Optimal Transport. In C. J. C. Burges, L. Bottou, M. Welling, Z. Ghahramani, & K. Q. Weinberger (Eds.), Advances in Neural Information Processing Systems (Vol. 26). Curran Associates, Inc. https://proceedings.neurips.cc/paper/2013/file/af21d0c97db2e27e13572cbf59eb343d-Paper.pdf
- Nguyen, J. P., Shipley, F. B., Linder, A. N., Plummer, G. S., Liu, M., Setru, S. U., Shaevitz, J. W., & Leifer, A. M. (2015). Whole-brain calcium imaging with cellular resolution in freely behaving Caenorhabditis elegans. Proceedings of the National Academy of Sciences of the United States of America, 9, 33. https://doi.org/10.1073/pnas.1507110112
- Venkatachalam, V., Ji, N., Wang, X., Clark, C., Mitchell, J. K., Klein, M., Tabone, C. J., Florman, J., Ji, H., Greenwood, J., Chisholm, A. D., Srinivasan, J., Alkema, M., Zhen, M., & Samuel, A. D. T. (2016). Pan-neuronal imaging in roaming Caenorhabditis elegans. In Proceedings of the National Academy of Sciences of the United States of America (Vol. 113, Issue 8). https://doi.org/10.1073/pnas.1507109113