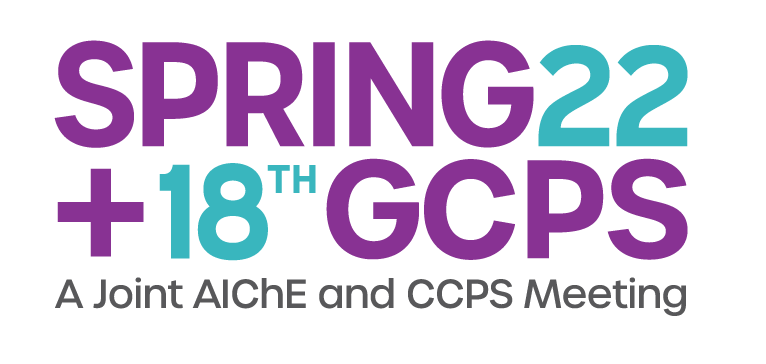
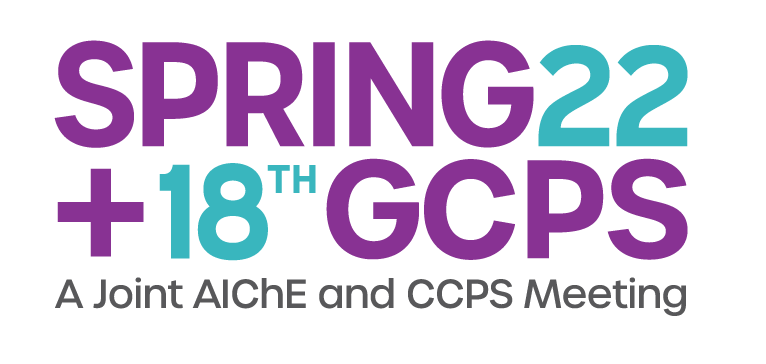
Until recently, a formulation scientist could do not much more than relying on experimental trial and error, perhaps assisted by robotic-assisted screening or ancient wisdom.
With a phenomenal technological advancement in AI-driven protein structure prediction by Googleâs Deepmind (1,2) and similar academic AI initiatives (3), we now suddenly have the possibility to generate structures on a (multiple-)proteome-wide scale. Perhaps only a few of those structures will be of atomic accuracy; that is the resolution one needs for small molecules drug discovery. But for many a formulation challenge, one does not need atomistic resolution: a relatively rough 3D structure, organized on the level of groups of atoms (âbeadsâ) could be enough. However, there is one challenge: in the translation of structure to formulation, one needs both atomic positions (albeit rough) and thermodynamics interactions.
It is precisely on the level of overlaying the AI-generated rough structure with coarse-grained (CG) modeling that one can hybridize data-driven and physics-based modeling into a new AI-CG hybrid algorithm. The AI method is determined by statistics, whereas the coarse-grained modeling relies on physics.
We showcase the AI-CG algorithm by a few examples where we take protein structures generated by Deepmind and then coarse-grain the structures with Simcenter Culgiâs Automated Fragmentation and Parameterization method. Once on the coarse-grained level, it is relatively easy to calculate, for example, the second virial coefficient or even to simulate the diffusion of a few coarse-grained protein molecules by Stokesian Particle Dynamics. The hybrid AI-CG algorithm takes only a few minutes, or at most a few hours, to execute on a modest PC., so still of sufficient efficiency for screening purposes.
- Jumper, J. et al.Naturehttps://doi.org/10.1038/s41586-021-03819-2 (2021).
- Tunyasuvunakool, K. et al.Naturehttps://doi.org/10.1038/s41586-021-03828-1 (2021).
- Baek, M. et al. Science, https://doi.org: 10.1126/science.abj8754 (2021)